Our Series A investment in Peptone
Machine learning is reimagining the drug discovery process—here’s why we’re backing the team that’s bringing order to disordered proteins.
A year ago, we published a roadmap we had been researching for years called Unlocking Machine Learning for Drug Discovery. Since then, the healthcare team at Bessemer has talked to hundreds of extraordinarily talented entrepreneurs who are leveraging machine learning to reimagine the ways in which drugs are discovered and developed. In talking to these entrepreneurs, we’ve never been more excited about the potential for new computational methods to expedite and industrialize many components of the drug discovery process.
One application we see as particularly promising is the potential for computational approaches to definitively predict the underlying structure of proteins, ultimately allowing for targeted and rational drug design processes against novel targets. In particular, a high-value area we’ve been evaluating is around representation learning for molecular and structural biology.
Our thesis is that novel machine learning model architectures that more accurately represent and generate biological structures, properties, and interactions with potential targets could identify more promising drug candidates.
Last year, we were fortunate to meet a team that’s been able to solve the “structure problem” associated with disordered proteins and regions. Kamil Tamiola, Matt Heberling, Ben Owens, Carlo Fisicaro, Bojana Popovic and the team at Peptone have set out to solve the limitations that exist from AlphaFold and other approaches to drug intrinsically disordered regions (IDPs). Today, we’re thrilled to announce our investment to co-lead Peptone’s Series A alongside our friends at F-Prime Capital, Walden Catalyst, dRx Capital, and Hoxton Ventures.
Unlocking a new landscape of protein targets
We’re intrigued by this opportunity because upwards of 53% of all proteins include mobile regions, known as intrinsically disordered regions (IDRs), or are completely intrinsically disordered proteins (IDPs). These disordered regions and proteins are often implicated in the pathogenesis and progression of disease across immunology, oncology and neurodegeneration. Yet, these disordered proteins have historically been nearly impossible to drug. They are poorly understood, difficult for traditional structure visualization techniques, such as X-ray crystallography and cryogenic electron microscopy, to experimentally recreate, and inherently have unstable binding pockets for potential drug candidates to target.
However, a company that is able to computationally solve and generate the structure and dynamics of these IDPs has the potential to unlock both a new landscape of protein targets that suddenly become druggable and a new drug class of biologics that contain intrinsically disordered regions themselves.
Last year, the biotech community galvanized around the potential for DeepMind’s AlphaFold 2 to “predict the 3D shape of proteins from their genetic sequence with, for the most part, pinpoint accuracy.” Nature magazine described these developments unfolding over the last 16 months as “AlphaFold Mania.”
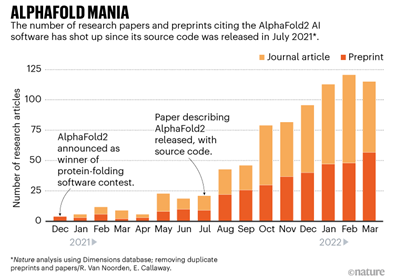
As promising as AlphaFold 2 is, significant limitations still exist particularly when it comes to disorder. AlphaFold’s algorithms rely heavily on known structures within the Protein Data Bank, which is primarily composed of proteins with fixed structures, so AlphaFold’s utility to-date is largely limited to ordered proteins where the 3D structure is stable.
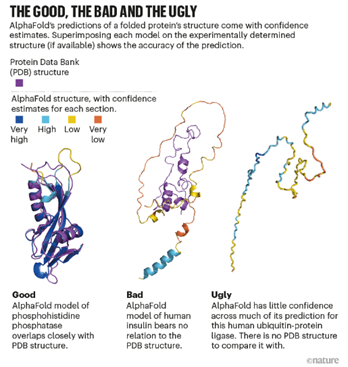
Peptone’s potential
The Peptone approach immediately stood out to us as the team had ventured on a new frontier to develop therapeutics against intrinsically disordered protein drug targets that have been largely considered undruggable by traditional drug discovery methods. Plus, Kamil, Matt, Ben, Carlo, Bojana and the team are uniquely “bilingual” in biotech, computer science, and physics (among many other languages!) making them especially suited to develop best-in-class approaches to drug these proteins.
Peptone’s computational physics-based Oppenheimer platform incorporates a proprietary supercomputer that leverages biophysics and AI/ML to identify the structure and dynamics of these difficult-to-drug disordered proteins with extraordinary resolution. This allows Peptone to then generate precise and realistic representations of protein behaviors and ultimately identify the most plausible target binding sites and therapeutic approaches against these important disease-causing proteins.
We believe that Peptone’s technology has vast potential to create many proprietary therapeutics to treat diseases with significant need, as well as to partner on co-development opportunities given their impressive proof-of-concept work. While this is just the beginning of Peptone’s story and there’s a lot of work ahead, we’re thrilled that the team has welcomed us along for the journey.
Forge ahead!